Introduction
Dating back to the 3rd century BC, the first instance of fraud was carried out by two Greek sea merchants who decided to sink their loaded cargo to enrich themselves. The plan failed – they were both caught in the act, and one lost his life trying to escape, while the other faced charges in the Athenian courts. (Source: https://www.fraud.com/post/the-history-and-evolution-of-fraud#The_first-ever_fraud_case)
Financial fraud is nothing new and each year, the tales of fraudulent activities are more and more incredible.
In recent times, Sam Bankman-Fried, the now-fallen “King of Crypto” comes to mind. Once hailed as the spokesperson for crypto in Washington DC, he now faces decades in prison. He had a cryptocurrency exchange called FTX, a trading firm called Alameda Research, and at his peak, a net worth of $26 billion, according to Bloomberg. However, he was arrested and charged with fraud after FTX collapsed into bankruptcy with billions being reported missing. He was accused of stealing billions through wire fraud and money laundering in what amounts to one of the largest financial crimes in US history. The central allegation was that he used customer funds to prop up his own risky investments in his trading firm, Alameda Research, and to fund an extravagant lifestyle.
Another cryptocurrency player, former Celsius Networks CEO Alexander Mashinsky was also charged by US prosecutors with defrauding customers and manipulating the price of the platform’s crypto token, CEL, while selling their own tokens at inflated prices. Celsius presented itself as a secure bank-like platform where customers could deposit crypto assets and earn interest. However, the indictment maintains that Mashinsky ran Celsius as a risky investment fund, misleading clients and making them unwitting investors in a venture that was much riskier and less profitable than represented.
According to Federal Trade Commission, consumers reported losing more than $10 billion to fraud in 2023 – the first time that fraud losses have reached this level. It indicates a 14% increase over reported losses in 2022. Most of the money was lost to investment scams: more than $4.6 billion, indicating a 21% increase over 2022. Imposter scams, with losses of nearly $2.7 billion came in second. However, consumers reported losing more money to bank transfers and cryptocurrency than all other methods combined. (Source: https://www.ftc.gov/news-events/news/press-releases/2024/02/nationwide-fraud-losses-top-10-billion-2023-ftc-steps-efforts-protect-public)
What Types of Businesses Need Protection?
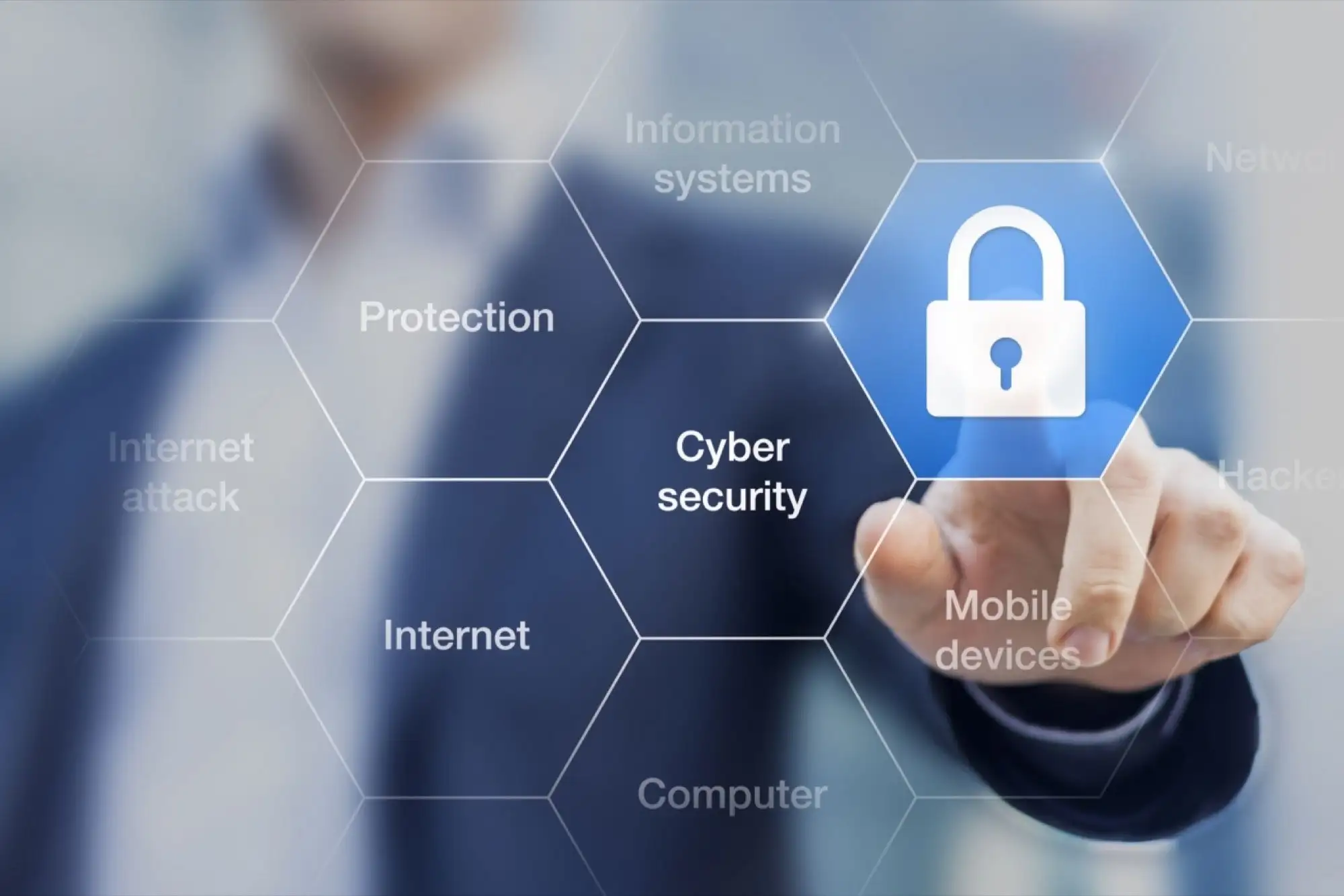
Image source: NicoElNino | Getty Images
69% of global executives and risk professionals expect the risk of fraud to increase over 2024 with cybersecurity and data breaches as the most prominent ones. Investments in technology, increase in cybersecurity budgets and frequent business risk assessments are the three main steps that firms intend to take during the next year to combat the increase in financial crime. (Source: Fraud and Financial Crime Report Can technology stop the threat of economic, crypto and ESG crimes? 2023)
Businesses that are most susceptible to fraud include:
- Banks and financial institutions: Primary targets for fraud, banks and FIs handle vast amounts of money and customer data, and therefore, are prone to unauthorized transactions and data breaches.
- Credit card companies: Fraud risk is high because of stolen card information and fraudulent transactions.
- E-commerce platforms: With the rise in online shopping, the possibility of payment fraud, identity theft, and phishing attacks is high. According to estimates, e-commerce companies lose up to 3% of annual revenue due to fraud.
- Investment firms: Targets for hacking and fraudulent schemes, investment firms have large sums and sensitive financial data with them.
- Cryptocurrency exchanges and wallet services: Due to their digital nature and anonymity, cryptocurrencies are ideal for fraudulent activity.
Because trust is a crucial factor in these businesses, and they are evolving continuously, the need for fraud detection becomes imperative. Traditional fraud detection systems are becoming less effective – since fraudulent activity is evolving simultaneously – and manual detection and prevention are extremely resource-intensive. This is where the role of artificial intelligence (AI) becomes significant in fraud detection and prevention.
Pro tip: Are you looking for a trusted partner to implement cost-effective AI-based fraud detection? Experts from Data Pilot are ready to advise and help! Contact us today.
How does AI fraud detection work?
The approach relies on machine learning (ML) to identify behavior patterns and potentially fraudulent activities in large datasets. The algorithms are trained to learn from historical data, recognize patterns and anomalies that indicate fraud. ML models improve with time because of learning from fresh data; they become more accurate predictors and can adjust to detect fraudulent activity. This proactive defense mechanism gives companies an effective tool to ensure security and integrity of transactions.
The beauty of ML is that it enables organizations to detect fraud more quickly and extensively.ML algorithms start by establishing a baseline of normal transaction patterns and user behaviors. The system is trained to monitor data regularly and pick up on any deviations. The more data the algorithms receive, the better they get at accurately detecting fraud. Over time, the AI model adjusts its settings in response to fresh and varied data, improving its ability to distinguish between suspicious and genuine activity.Feature extraction is the second step in developing an ML model, which identifies authentic and fraudulent customer behavior patterns. For example, the consumers’ identities, locations, networks, orders, and preferred payment methods are recorded.
Depending on how sophisticated the fraud detection system is, a different set of features will be extracted every time.The next step involves training an ML algorithm with a set of rules through a training process so that it can differentiate between real and fraudulent transactions. After the training is over, it generates a machine learning system that is more adept at detecting fraudulent activities. In a shorter length of time, the algorithm can accurately identify frauds.
How is AI used in fraud detection
- Automated anomaly detection: Essentially a way to find patterns that deviate from the norm. Such patterns include unusual transaction amounts, multiple transactions from the same device, or purchases made from different locations in a short time frame. When the AI notices a discrepancy, it will detect and mark the transaction for additional examination.
- Behavioral analysis: Examines user patterns to identify anomalies that might indicate fraudulent activity. For example, any purchases outside of the usual transactions can be flagged as suspicious.
- Natural Language Processing (NLP): Analyzes the vast amount of textual data generated in financial transactions, such as emails, customer chats and documents. NLP uses sentiment analysis to identify language patterns in communication, enables verification by checking key entities as names, locations, and categorizes text data as legitimate requests or spam. For instance, any customer changing their account information and then sending an email requesting a password reset, can be seen as a potential fraud attempt by AI.
- Continuous learning: New data can be used to train AI algorithms, increasing their efficacy and accuracy over time. With this ongoing learning, fraud detection systems are kept at par with the most recent techniques and trends in fraud.
Implementing AI-based fraud detectio
- JPMorgan Chase
An interesting use case, JPMorgan Chase has successfully leveraged the power of machine learning for fraud detection. AI algorithms analyze transaction patterns, user behavior, and historical data, resulting in a significant reduction in false positives and improved accuracy of fraud detection.
- PayPal
PayPal is a pioneer in online payments. It has enabled behavioral analytics powered by AI to strengthen its fraud detection capabilities. By analyzing existing data on user behavior, transaction histories, and so on, the AI system builds a baseline profile of each user’s activities. As a result, the AI system can flag anomalies, and prevent unauthorized transactions.
- Capital One
Capital One, a leading financial institution, has leveraged machine learning to create a dynamic and adaptive fraud prevention system. Algorithms continuously analyze transaction data and user behavior to identify patterns indicative of fraud.
- Mastercard
Mastercard has incorporated AI-driven biometric authentication to enhance security and user experience. Facial recognition and fingerprint scanning technologies ensure that only authorized individuals can initiate transactions, thus preventing identity theft.
Source: (https://www.linkedin.com/pulse/case-studies-how-ai-based-fraud-detection-has-helped-fravf/)
The Bottom Line
There is no doubt that AI technologies have revolutionized the landscape of fraud detection by providing tools that are not only faster but more accurate than traditional methods. Machine learning algorithms analyze vast datasets to identify patterns and anomalies that might indicate fraudulent activity. The best part is that AI-powered systems adapt over time, continually improving their detection capabilities as they learn from new data. Ultimately, the integration of AI in fraud detection is indispensable for organizations seeking to protect their assets and maintain trust in an increasingly digital world.
By Tooba Shah.